Leveraging AI to Transform Scientific Research
AI is transforming the processing, analysis, and utilisation of data in scientific research, technology, and the social sciences. However, this advancement comes with inherent risks. To ensure responsible and effective use, robust ethical guidelines, greater transparency, and improved data curation are essential.
Key Themes and Insights
1. The Promise of AI in Research
- AI is revolutionising the way researchers process and analyse large data sets, significantly accelerating the pace of discoveries.
- Applications span multiple fields:
- In life sciences, AI aids in protein folding analysis, drug discovery, and genomics.
- In Earth sciences, it helps model climate change and predict environmental disasters.
- Social sciences benefit from AI-driven sentiment analysis and behavioural studies.
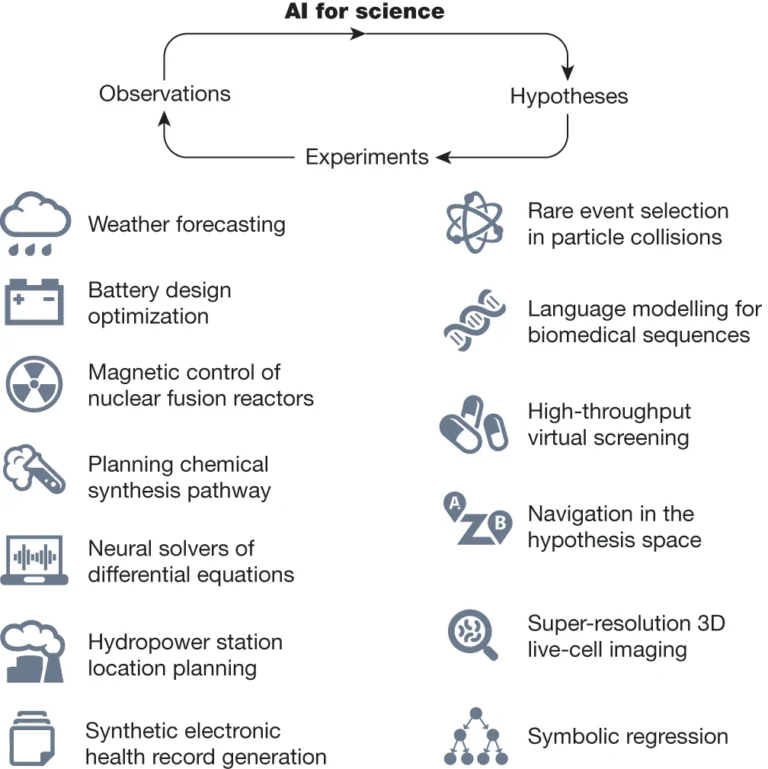
2. Challenges and Risks
- Data Bias and Fairness
- AI systems often amplify biases present in their training data, potentially leading to discriminatory outcomes.
- Examples include biased algorithms in healthcare that underperform for certain racial or socioeconomic groups.
- Lack of Transparency
- Many AI tools operate as “black boxes,” making it difficult to trace the logic behind their decisions.
- This lack of explainability can undermine trust in AI-driven findings.
- Over-Reliance on Automation
- The increasing automation of research processes risks diminishing critical thinking and creativity among researchers.
3. Ethical Guidelines and Governance
- The document underscores the importance of establishing robust governance frameworks to manage AI’s use in research.
- Ethical principles should include:
- Transparency: Clear documentation of data sources, methodologies, and assumptions.
- Equity: Ensuring AI benefits diverse populations and avoids perpetuating inequality.
- Accountability: Human oversight must remain central to AI-driven research to ensure accountability for decisions and outcomes.
4. The Importance of Data Curation
- High-quality, well-curated data sets are crucial for the reliability of AI models.
- Collaborative repositories adhering to FAIR principles (Findable, Accessible, Interoperable, Reusable) should be prioritised to support robust AI research.
5. Interdisciplinary Collaboration
- Successful integration of AI into research requires collaboration across disciplines.
- The article advocates for partnerships among data scientists, domain experts, policymakers, and ethicists to address the complex challenges of AI governance.
6. Call to Action
- Researchers and institutions must proactively adopt guidelines that promote responsible AI use.
- Funding agencies, universities, and publishers should enforce standards that emphasise data quality, ethical considerations, and inclusivity.
Conclusion
The integration of AI into research presents a double-edged sword. While it offers unprecedented opportunities to revolutionise data analysis and discovery, it also introduces risks of bias, lack of transparency, and ethical dilemmas. Addressing these challenges requires a collective effort to implement ethical standards, promote transparency, and ensure equitable benefits from AI technologies. By prioritising collaboration and responsible practices, the research community can harness AI’s full potential while safeguarding the integrity of scientific inquiry.