Generative AI Hype Diminishes, Ushering in Practical Applications
Less than two years after the launch of ChatGPT ignited widespread excitement about generative AI, initial expectations of a technological revolution have tempered. Early predictions, such as Goldman Sachs’ forecast of 300 million job disruptions due to AI, have not materialised as anticipated. Many AI-driven projects, including McDonald’s automated drive-through ordering system, have been discontinued following operational challenges.
This trajectory aligns with the Gartner hype cycle, which outlines a progression from inflated expectations to a trough of disillusionment, eventually leading to practical productivity. A recent Gartner report indicates that most generative AI technologies are currently at the peak of inflated expectations, with full productivity expected in two to five years.
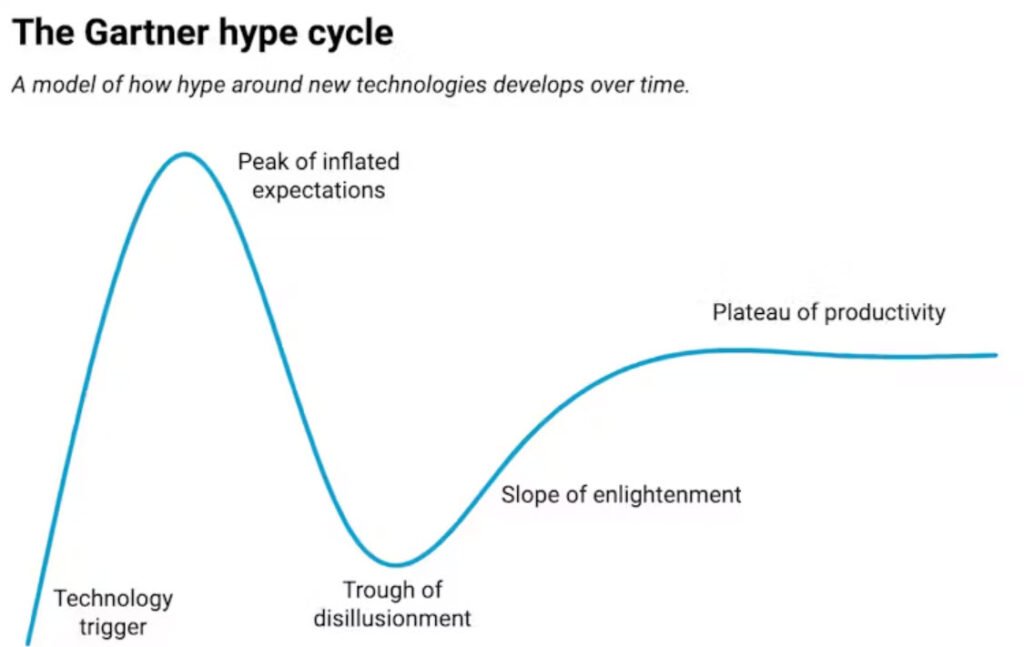
Key Points
- High Failure Rate: A study by the RAND Corporation reveals that 80% of AI projects do not succeed, highlighting challenges in implementation.
- Performance Inconsistencies: Generative AI systems exhibit the ability to solve complex tasks yet struggle with simpler ones, complicating assessments of their reliability.
- Shift in Adoption Strategies: Businesses are increasingly utilising AI to support human workers rather than replace them, focusing on efficiency and product quality improvements.
- Emergence of Smaller Models: There’s a growing trend towards developing smaller, cost-effective generative AI models tailored to specific tasks, enhancing accessibility and reducing expenses.
As the initial hype subsides, the focus is shifting towards integrating AI in ways that complement human capabilities, paving the way for more sustainable and effective applications.